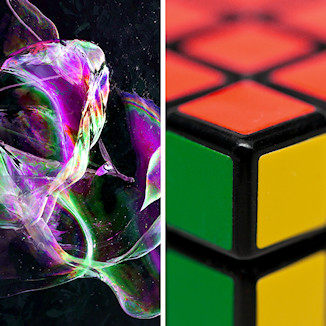
Getting Your Data Ready for AI Workloads
According to Forbes, 64% of businesses anticipate that AI will enhance productivity, showcasing growing confidence in its transformative potential. However, poor data quality remains a significant barrier to fully realizing AI’s benefits.
Data silos, hyper-segmentation, and inconsistent information can result in inaccurate AI predictions. To train effective AI models, it’s crucial to ensure high-quality, clean data.
Additionally, strict compliance with privacy regulations is essential when using customer data for AI. Managing large datasets securely is vital, as it increases the risk of data breaches. Customer concerns about data misuse can also undermine trust in AI systems.
Low-quality data may lead to AI interactions that feel impersonal or erroneous, heightening customer frustration. Therefore, balancing AI automation with human oversight is necessary to address shortcomings.
Key Discussion Points:
1. While achieving perfect data quality is unrealistic, setting and meeting a company-approved data quality standard is attainable.
2. Strategies for mitigating the impact of poor data quality on AI-driven decision-making.
3. How Data Intelligence tools can help identify and manage PII/PHI data across your landscape.