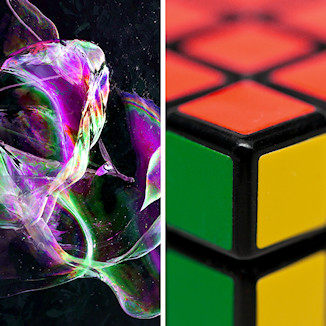
Verification of Quantum Neural Networks for Time Series Forecasting
This abstract describes the innovative application of Quantum Computing (QC) in enhancing the robustness of time series forecasting, particularly in the context of vehicle dynamics prediction. Ensuring the correctness of classical machine learning in this application poses significant challenges, primarily due to the high computational demand required for formally verifying the robustness of predictions against input perturbations. This task becomes increasingly complex with the dimensionality of input data, as it necessitates a vast number of samples to ensure reliability, making it both time-consuming and resource-intensive.
In addressing these challenges, the collaboration between the Fraunhofer Institute for Cognitive Systems IKS and Volkswagen Group Innovation (VW) showcases a groundbreaking approach by leveraging Quantum Neural Networks (QNNs). The project focuses on the dynamic behavior prediction of vehicles through the analysis of sensor data, integrating advanced input augmentation techniques and exploring the effects of minor changes in input data within a constrained Hamming distance. Unlike traditional methods, QC allows for the probabilistic verification of input sample robustness over a specified perturbation range, achieving this with far fewer number of predictions.
The utilization of QNNs, acting as an oracle, alongside the Grover search algorithm, offer a quadratic speed-up in estimating the frequency of accurate predictions for the original input class. This approach not only surpasses classical computational limits but also opens new avenues for enhancing the reliability and efficiency of time series forecasting in automotive and other complex systems. Through this initiative, Fraunhofer IKS and VW pave the way for innovative quantum solutions in real-world applications, heralding a new era in predictive analytics.