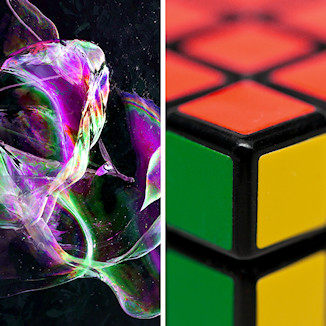
AI Systems Engineering: How AI and Engineering can go Hand in Hand
Using AI in productive environments is more than just building ML models. Especially in engineering contexts, it is important that any AI application fits into the users' work processes. Unlike with generative AI, the focus here is usually not on the creativity of the solution but instead on the reliability and trustworthiness of the AI-based solution. To this end, we highlight the advantages of AI Systems Engineering, the aim of which is to make AI and ML methods systematically usable according to the typical requirements and procedures of engineers. As an example of this approach, we show the application of ML methods in the domain of fatigue strength estimation. Fatigue strength estimation is a costly manual material characterisation process in which state-of-the-art approaches follow a standardised experiment and analysis procedure. In our application, we examine a modular, Machine Learning-based approach for fatigue strength estimation that will likely reduce the number of experiments and, thus, the overall experimental costs.